Improving Neural Machine Translation Models With Monolingual Data
Sennrich et al. Improving Neural Machine Translation Models with Monolingual Data.
Pdf Improving Neural Machine Translation Models With Monolingual Data Semantic Scholar
Improving Neural Machine Translation Models with Monolingual Data.
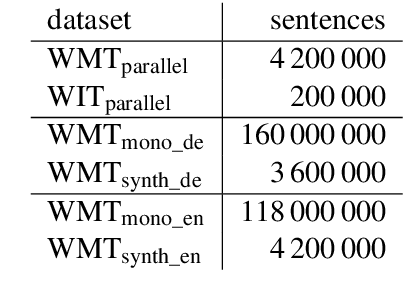
. ACL 2016 Rico Sennrich Barry Haddow Alexandra Birch. Improving neural machine translation NMT models using the back-translations of the monolingual target data synthetic parallel data is currently the state-of-the-art approach for training improved translation systems. Target-side monolingual data plays an important role in boosting.
Improving Neural Machine Translation Models with Monolingual Data. TitleImproving Neural Machine Translation Models with Monolingual Data. AbstractNeural Machine Translation NMT has obtained state-of-the art performancefor several language pairs while only using parallel data for training.
Sennrich R Haddow B Birch A 2016 Improving Neural Machine Translation Models with Monolingual Data. Under this framework we leverage large monolingual corpora to improve the NAR models performance with the goal of transferring the AR models generalization ability while preventing overfitting. Target-side monolingual data plays an important role in.
Bahdanau et al 2015 the decoder is es-. In contrast to previous work which combines NMT models with separately trained language models we note that encoder-decoder NMT architectures already have the capacity to. At the same time in recent years researchers have discovered that deep learning DL has solved the problems of semantic understanding errors and poor.
TitleImproving Neural Machine Translation Models with Monolingual Data. A UNMT model. On top of a strong NAR.
Back-translation is a critical component of Unsupervised Neural Machine Translation UNMT which generates pseudo parallel data from target monolingual data. Target-side monolingual data plays an important role in boosting fluency for phrase. Firstly proposes back-translation for monolingual data augmentation in neural machine translation tasks.
This paper propose new data augmentation they call back-tranlsation. Neural Machine Translation NMT has obtained state-of-the art performance for several language pairs while only using parallel data for training. We also show that.
86-96 54th Annual Meeting of the Association for. Monolingual data plays an important role in boosting fluency. In Proceedings of the 54th Annual Meeting of the Association for Computational Linguistics.
While the naive back-translation approach improves the translation performance substantially we notice that its. Improving neural machine translation models NMT with monolingual data has aroused more and more interests in this area and back-translation for monolingual data augmentation Sennrich et al. Submitted on 20 Nov 2015 v1 last revised 3 Jun 2016 this version v4 Neural Machine Translation NMT has obtained state-of-the art performance for several language pairs while only using parallel data for training.
Target-side monolingual data plays an important role in boosting fluency for phrase-based statistical machine translation and we investigate the use of monolingual data for NMT. In attentional encoder-decoder architectures for neural machine translation Sutskever et al 2014. Neural Machine Translation MT has radi-cally changed the way systems are developed.
The amount of available monolingual data in the target language typically far exceeds the amount of parallel data and models typically improve when trained on more data or data more similar to the translation task. Able monolingual data in the target language typi-cally far exceeds the amount of parallel data and models typically improve when trained on more data or data more similar to the translation task. TitleImproving Neural Machine Translation Models with Monolingual Data.
Given the parallel data and the target monolingual data they firstly train a backward NMT model ie from the target language to the source language on the parallel corpus and then use the learned model to translate the target. Target-side monolingual data plays an important role in boosting fluency for phrase-based statistical machine translation and we investigate the use. Target-side monolingual data plays an important role in.
Improving Neural Machine Translation Models with Monolingual Data. While Phrase-Based MT can seamlessly integrate very large language models trained on billions of sen-. This is a brief summary of paper for me to study and organize it Improving Neural Machine Translation Models with Monolingual Data Sennrich et al ACL 2016 that I read and studied.
Long Papers Association for Computational Linguistics ACL Berlin Germany pp. Target-side monolingual data plays an important role in boosting fluency for phrase-based statistical machine translation and we investigate the use. Neural Machine Translation NMT has obtained state-of-the art performance for several language pairs while only using parallel data for training.
Neural Machine Translation NMT has obtained state-of-the art performance for several language pairs while only using parallel data for training. Target side monolingual data plays an important role in boosting fluency for phrase based machine translation. 2016 has been taken as a promising development recently.
3 NAR-MT with Monolingual Data Augmenting the NAR training corpus with mono-lingual data provides some potential beneļ¬ts. Neural Machine Translation NMT has obtained state-of-the art performance for several language pairs while only using parallel data for training. AuthorsRico Sennrich Barry Haddow Alexandra Birch.
By pairing monolingual training data with an automatic backtranslation we can treat it as additional parallel training data and we obtain substantial improvements on the WMT 15 task English German 2837 BLEU and for the low-resourced IWSLT 14 task TurkishEnglish 2134 BLEU obtaining new state-of-the-art results. Improving Neural Machine Translation Models with Monolingual Data. AuthorsRico Sennrich Barry Haddow Alexandra Birch.
However since neural network machine translation NNMT only uses neural networks to convert between natural languages it also has shortcomings while simplifying the translation process. Improving Neural Machine Translation Models with Monolingual Data. Firstly we allow more data to be translated by the AR teacher so the NAR model can see more of the AR translation outputs than in the original train-ing data which helps the NAR model generalize better.
A major difference with the previous gener-ation Phrase-Based MT is the way mono-lingual target data which often abounds is used in these two paradigms. Non-autoregressive NAR neural machine translation is usually done via knowledge distillation from an autoregressive AR model. AbstractNeural Machine Translation NMT has obtained state-of-the art performancefor several language pairs while only using parallel data for training.
Neural Machine Translation NMT has obtained state-of-the art performance for several language pairs while only using parallel data. The quality of the backward system which is trained on the available parallel data and used for the back-translation has been shown in.
Pdf Improving Neural Machine Translation Models With Monolingual Data Semantic Scholar
Pdf Improving Neural Machine Translation Models With Monolingual Data Semantic Scholar
Neural Machine Translation Model With Attention Mechanism Download Scientific Diagram
Pdf Improving Neural Machine Translation Models With Monolingual Data Semantic Scholar
No comments for "Improving Neural Machine Translation Models With Monolingual Data"
Post a Comment